Motion Estimation by Deep learnIng in 2D eChocardiogrAphy: synthetIc Dataset and validation
This is a joint venture between CREATIS and Philips Research Suresnes FRANCE.
Overview
Motion estimation in echocardiography plays an important role in the characterisation of cardiac function, allowing the computation of myocardial deformation indices. However, there exists limitations in clinical practice, particularly with regard to the accuracy and robustness of measurements extracted from images. This can be explained by the variation in image quality between patients and by the presence of various artefacts inherent to the imaging modality.
Objectives
We propose a novel deep learning solution for motion estimation in echocardiography. Our network corresponds to a modified version of PWC-Net which achieves high performance on ultrasound sequences. In parallel, we designed a novel simulation pipeline allowing the generation of a large amount of realistic B-mode sequences with the possibility of adding reverberation artifacts.
Main contribution
The main contributions achieved in this project are :
- To overcome the problem of limited synthetic data in number and diversity, we created a new pipeline to generate large-scale synthetic ultrasound sequences with a wide range of cardiac deformations. Two types of synthetic data were thus generated, with and without reverberation artifacts;
- We showed that the PWC-Net architecture has the potential to produce relevant results on ultrasound images thanks to an adapted transfer learning procedure. This allows a better generalization of the network and a significant improvement of the results on clinical data;
- We further improve the performance of this network on ultrasound data by modifying its architecture to enhance its multi-scale analysis capability;
- We performed a thorough study of several temporal strategies that can be used to improve results during both the training and inference phases.
- We conducted the first study on the generalization of deep learning algorithms for motion estimation in echocardiography using a multi-center, multi-vendor and multi-disease dataset of real patients.
Open access synthetic dataset
Personalized pipeline
An overview of the workflow of our simulation pipeline is given below.
Some examples of the simulated sequences without and with reverberation artifacts. Don't hesitate to download each animation by right clicking on it for a better visualization.
Virutal patient 1
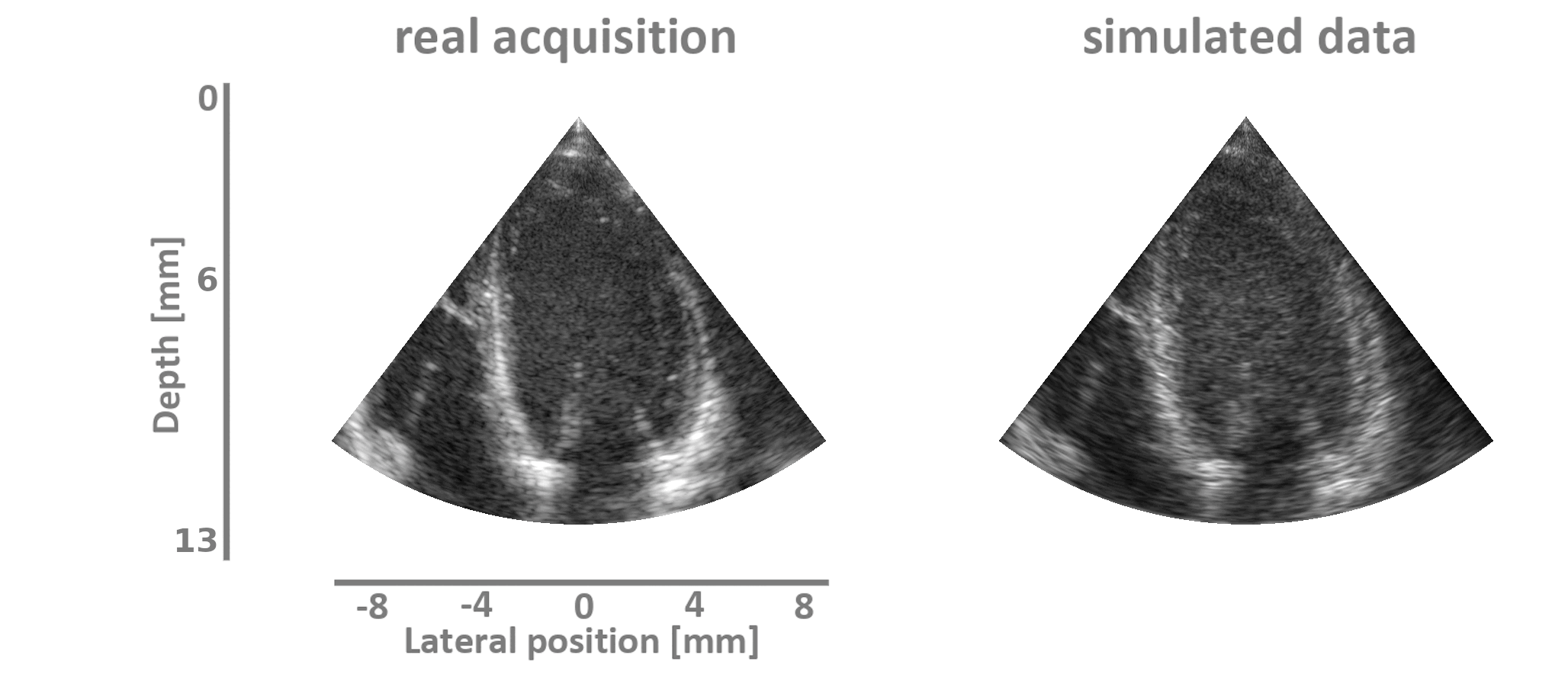
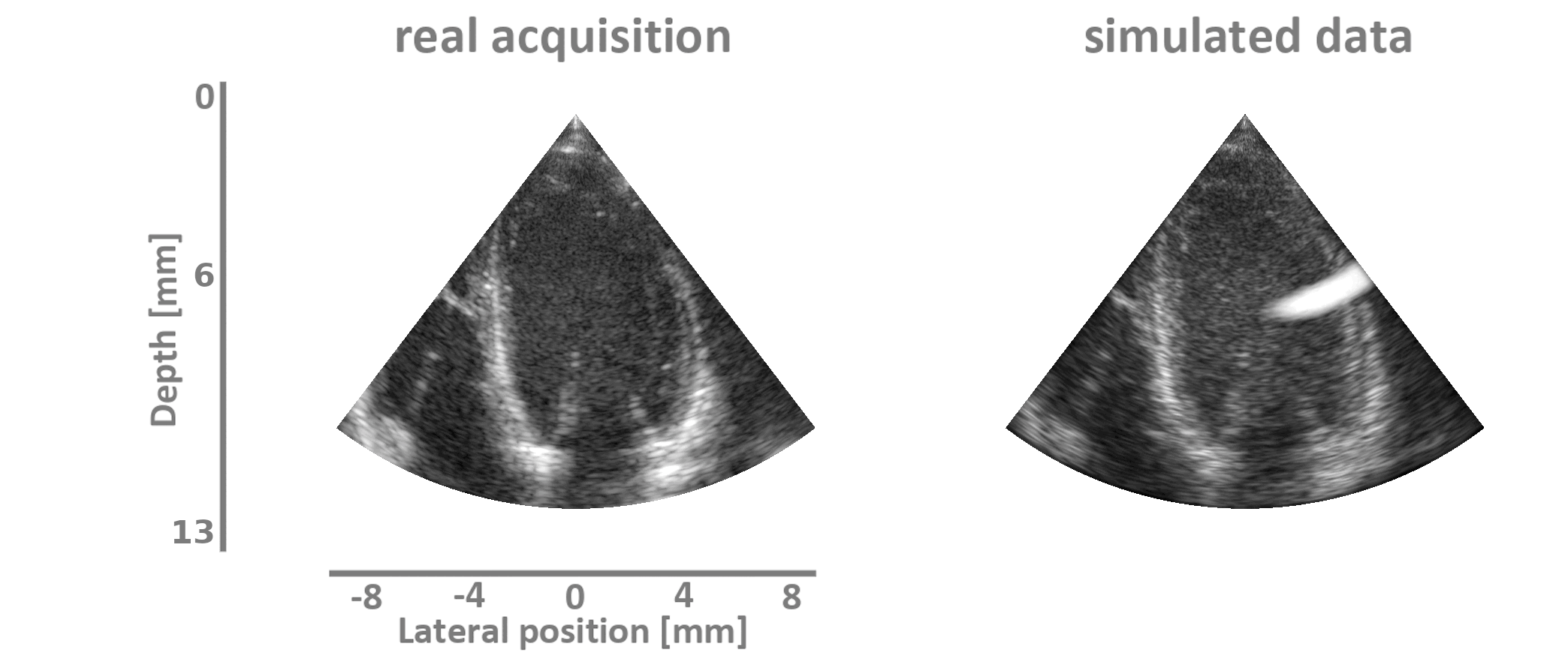
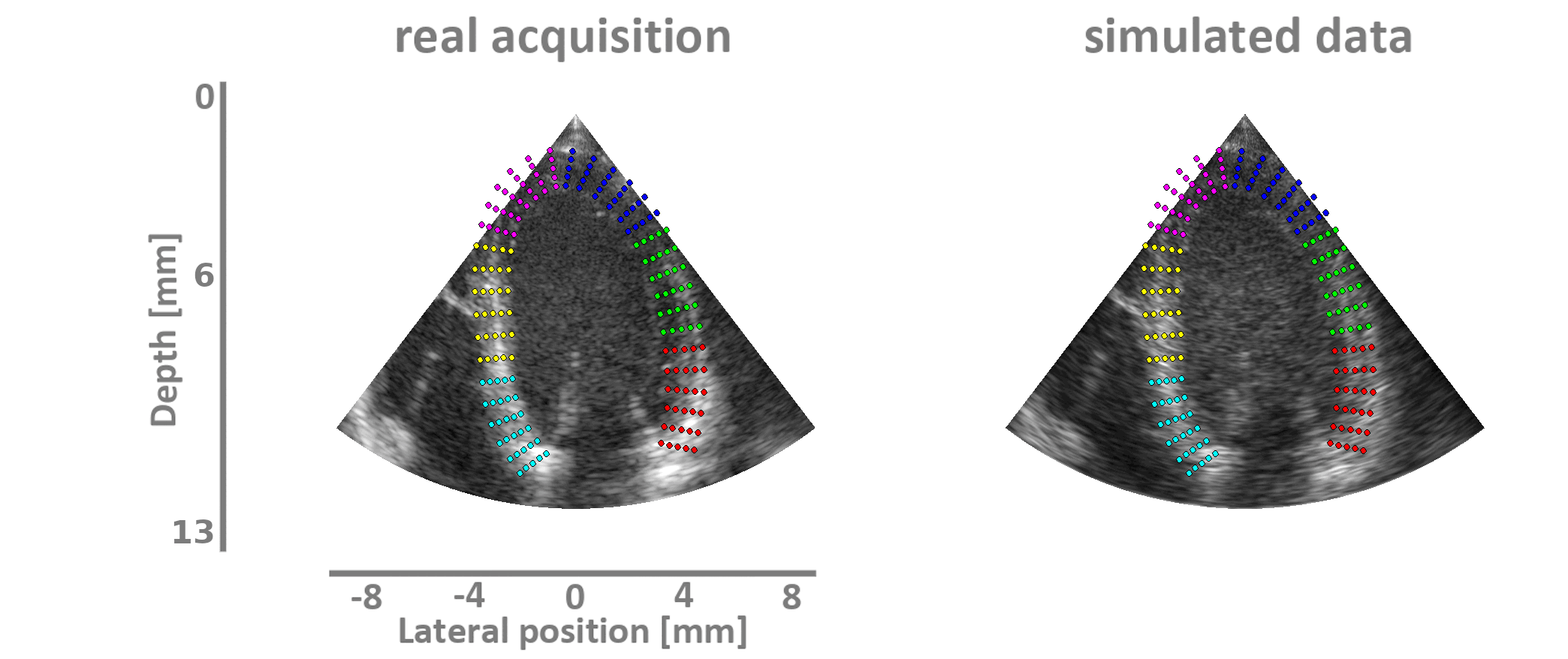
Virutal patient 2
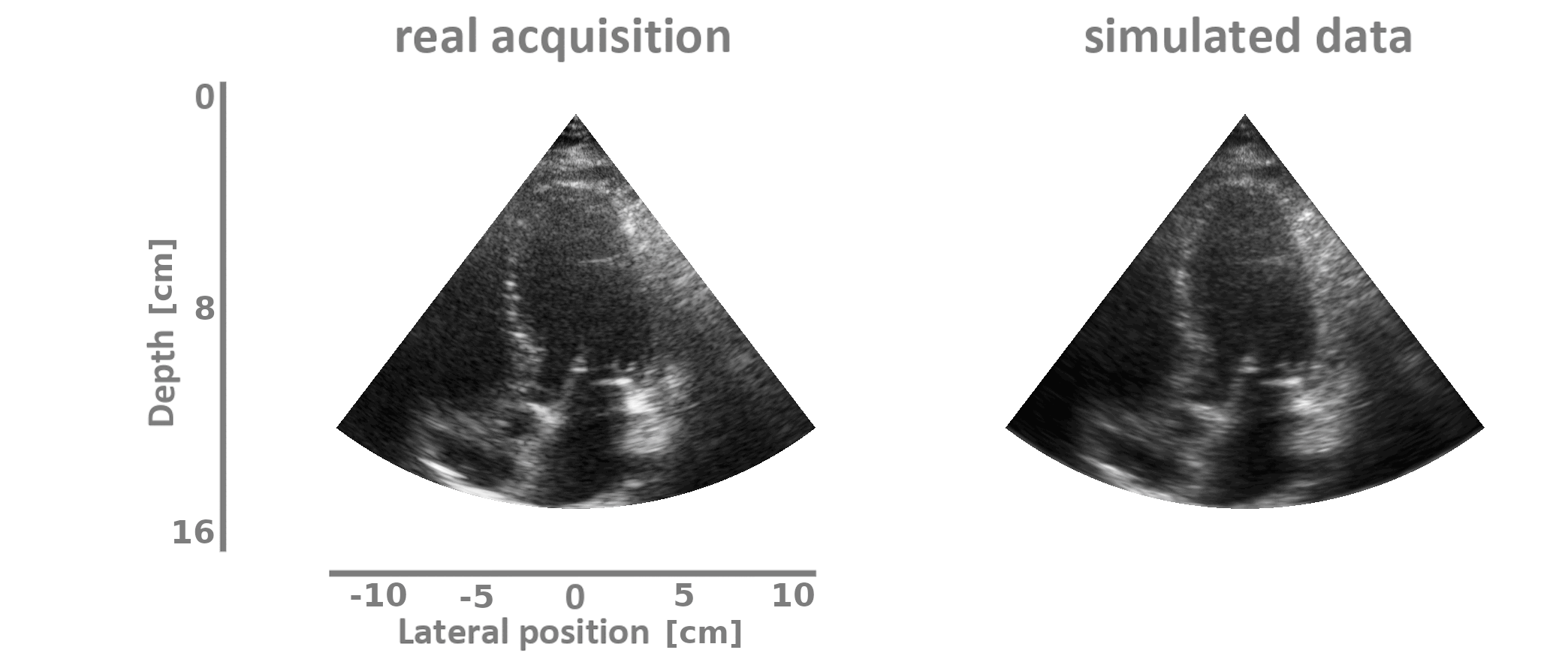
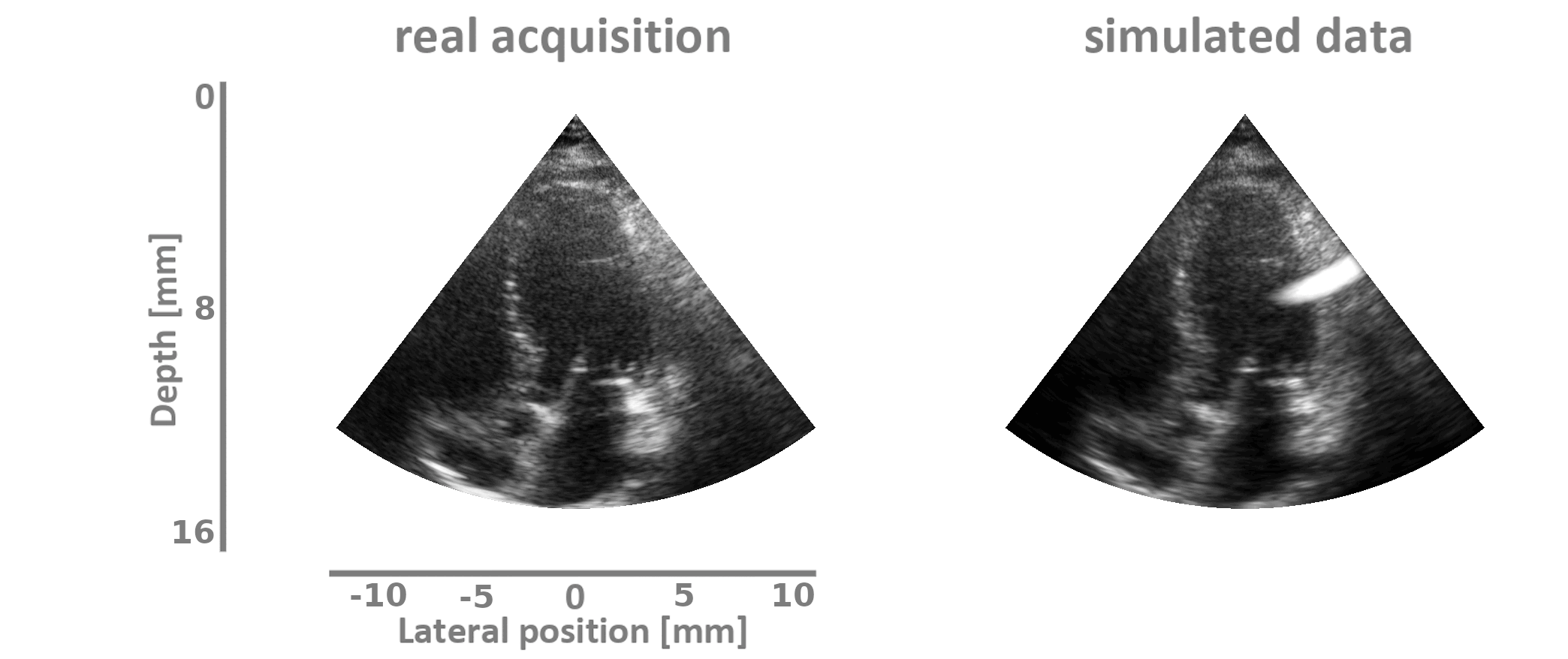
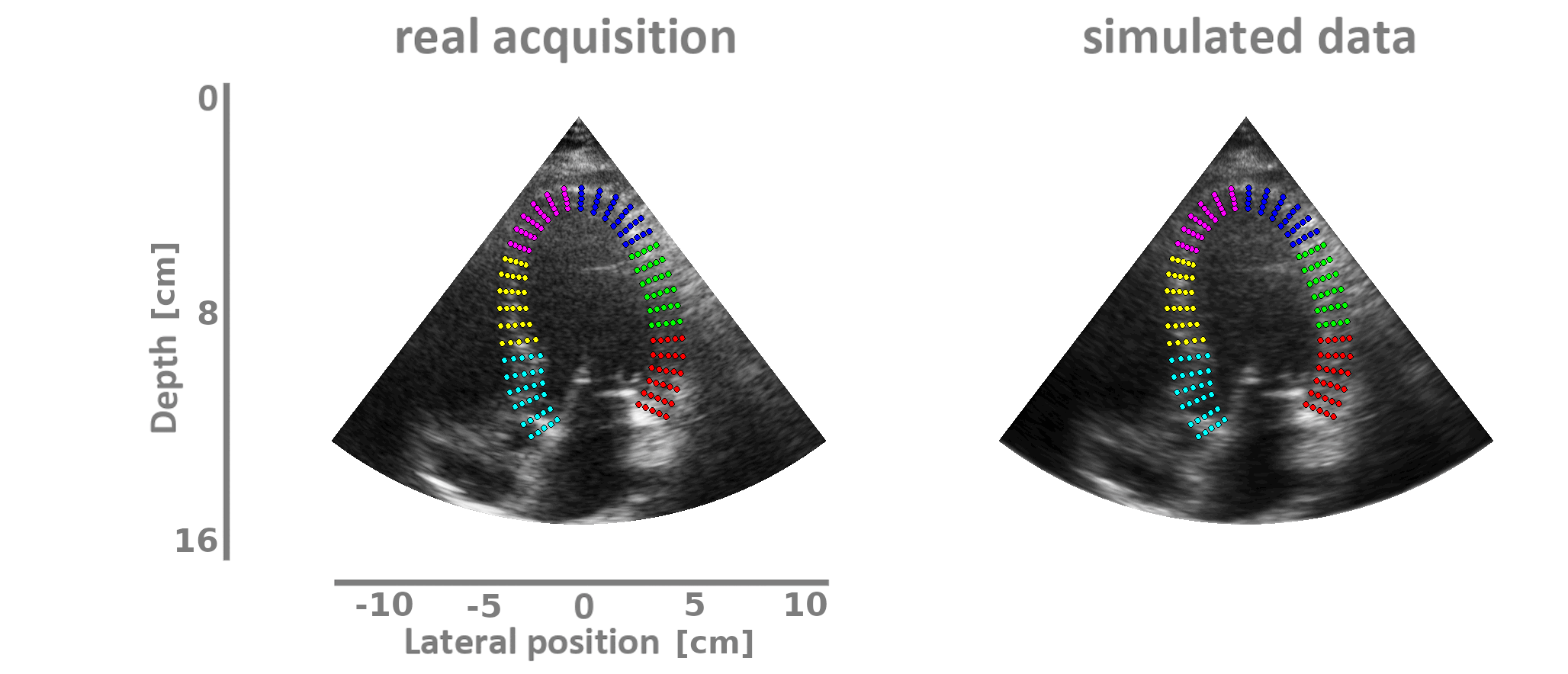
Get Started
To browse through the image database, simply connect to the syntheticCAMUS database, explore and download the images of interest or the entire collection. This database is public, so no login is required.
Motion estimation with deep learning
Overall architecture
Results on datasets of real patients
For all animations, the displayed contour corresponds to the one estimated by our method and the colorbar provides information on the error calculated from the reference contour drawn by an expert cardiologist..
Results from the GE dataset (CAMUS)
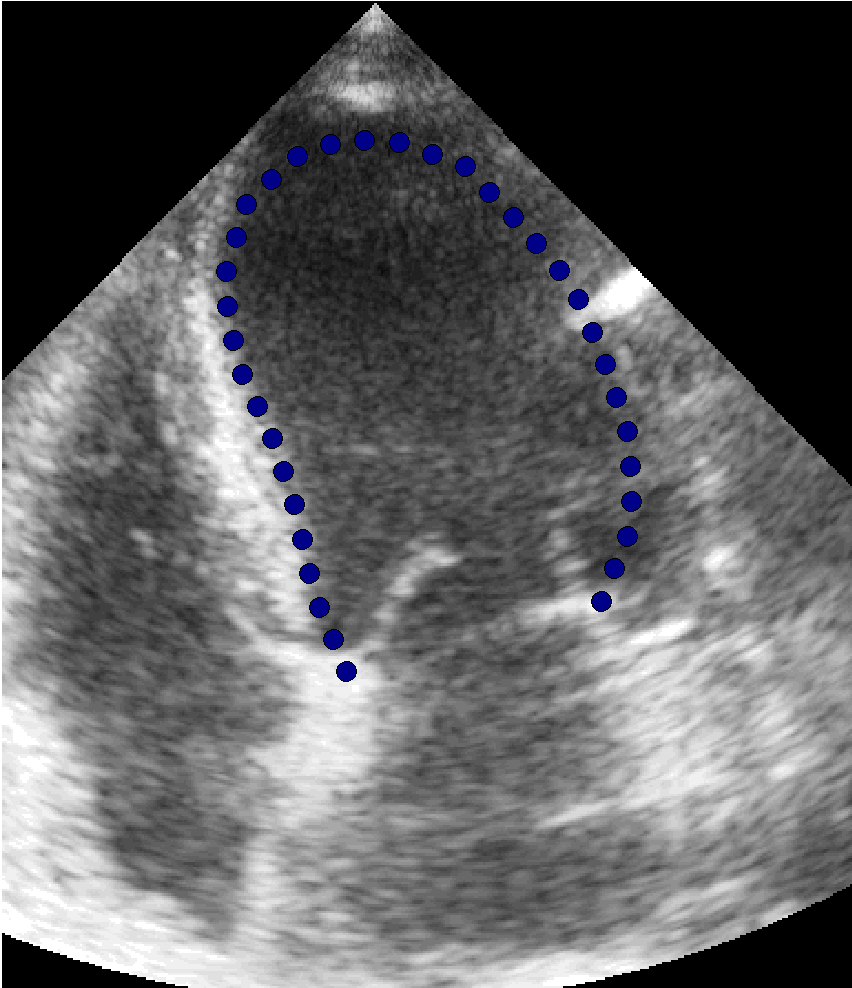
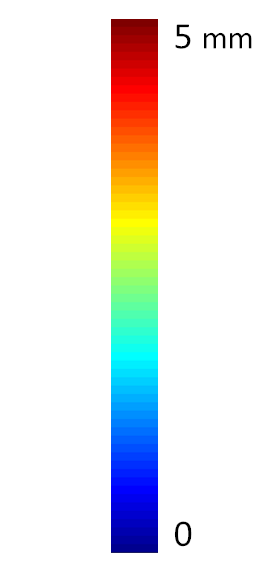
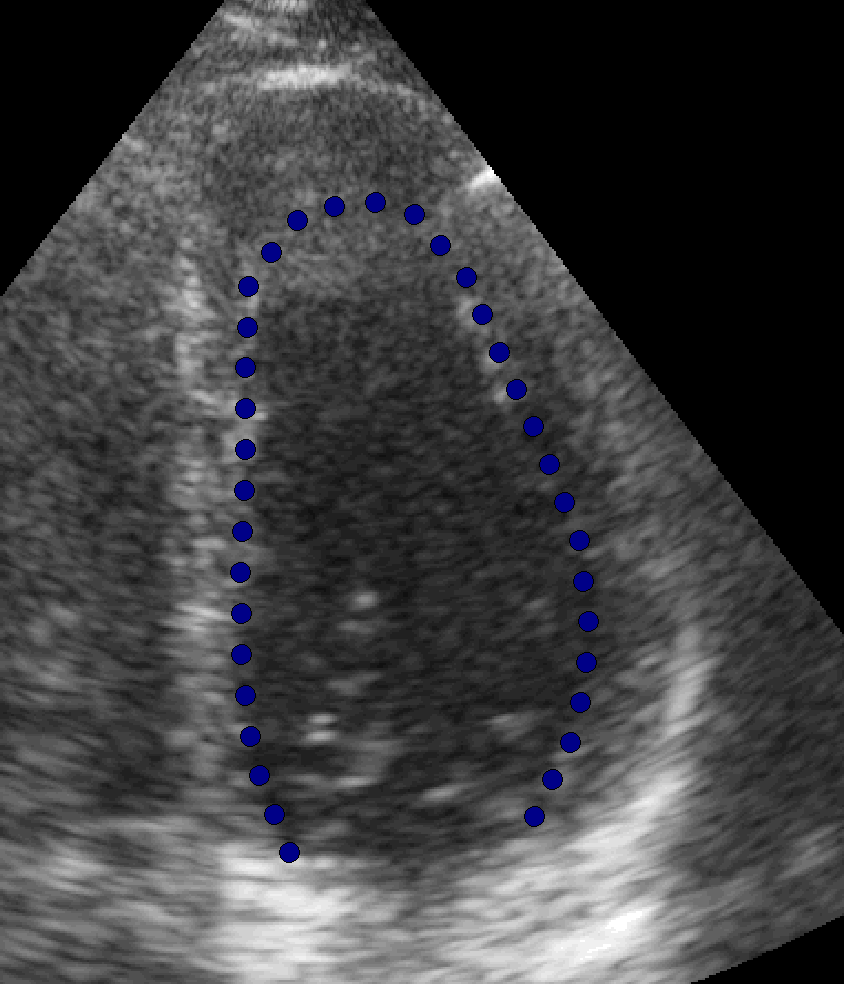
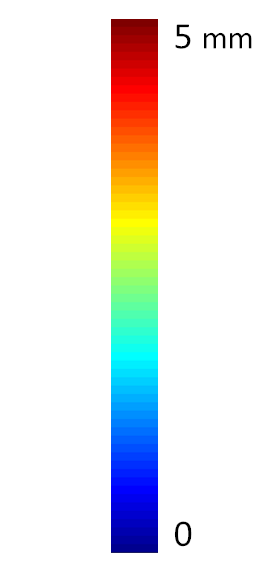
Results from the Philips dataset
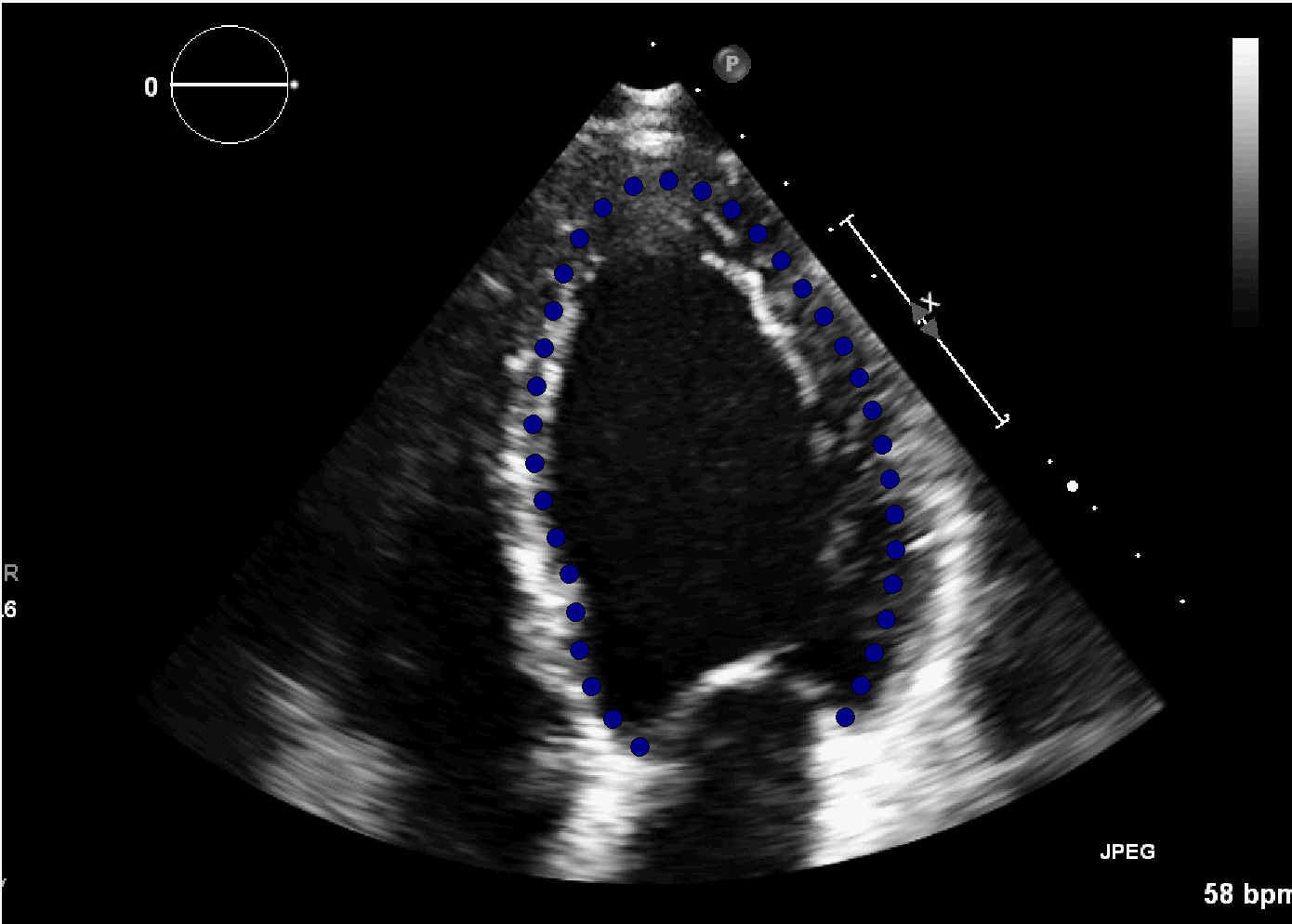
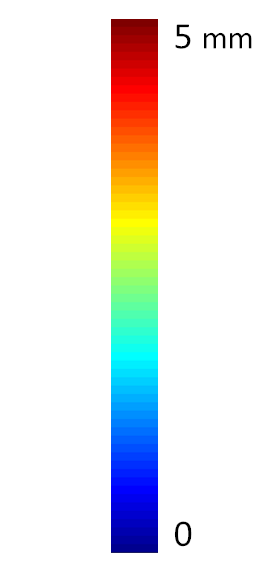
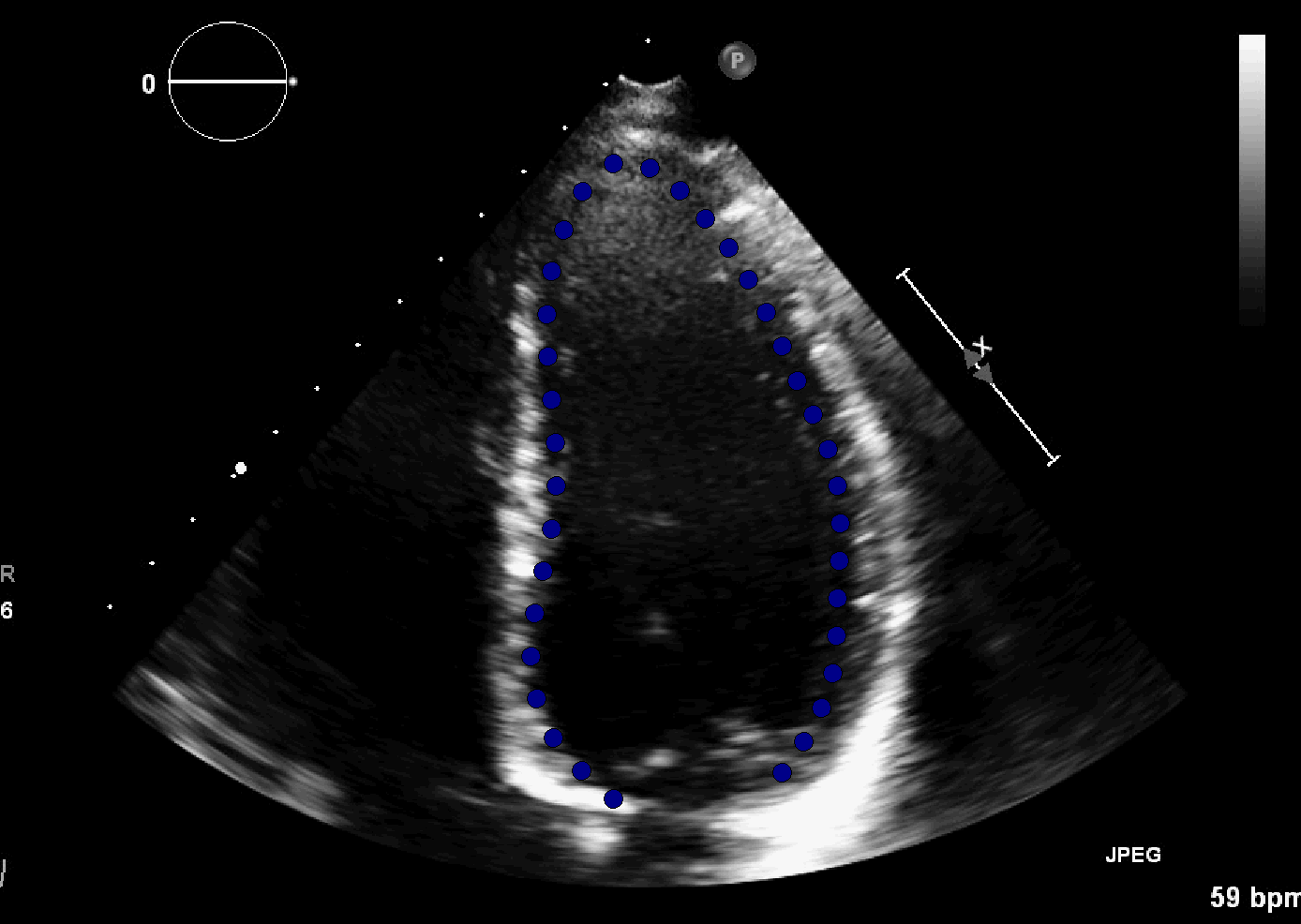
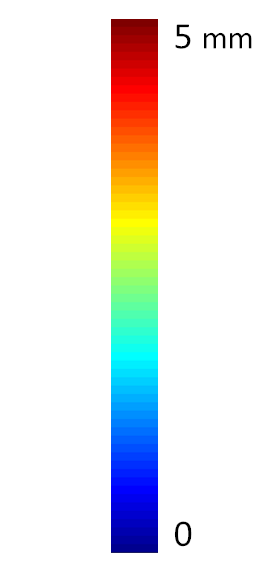
R&D Team
Ewan EVAIN | PhD student, Philips Research, Suresnes, France
|
Yunyun SUN | PhD student, CREATIS, France
|
Khuram FARAZ | Postdoctoral fellow, CREATIS, France
|
Damien GARCIA | Researcher at INSERM Institut, CREATIS, France
|
Eric Saloux | Cardiologist at the University Hospital of Caen, CHU Caen, France
|
Bernhard L. Gerber | Cardiologist at Cliniques Universitaires Saint-Luc UCL, Brussels, Belgium
|
Mathieu De Craene | Research Engineer, Philips Research, Suresnes, France
|
Olivier BERNARD | Full professor, CREATIS, France
|